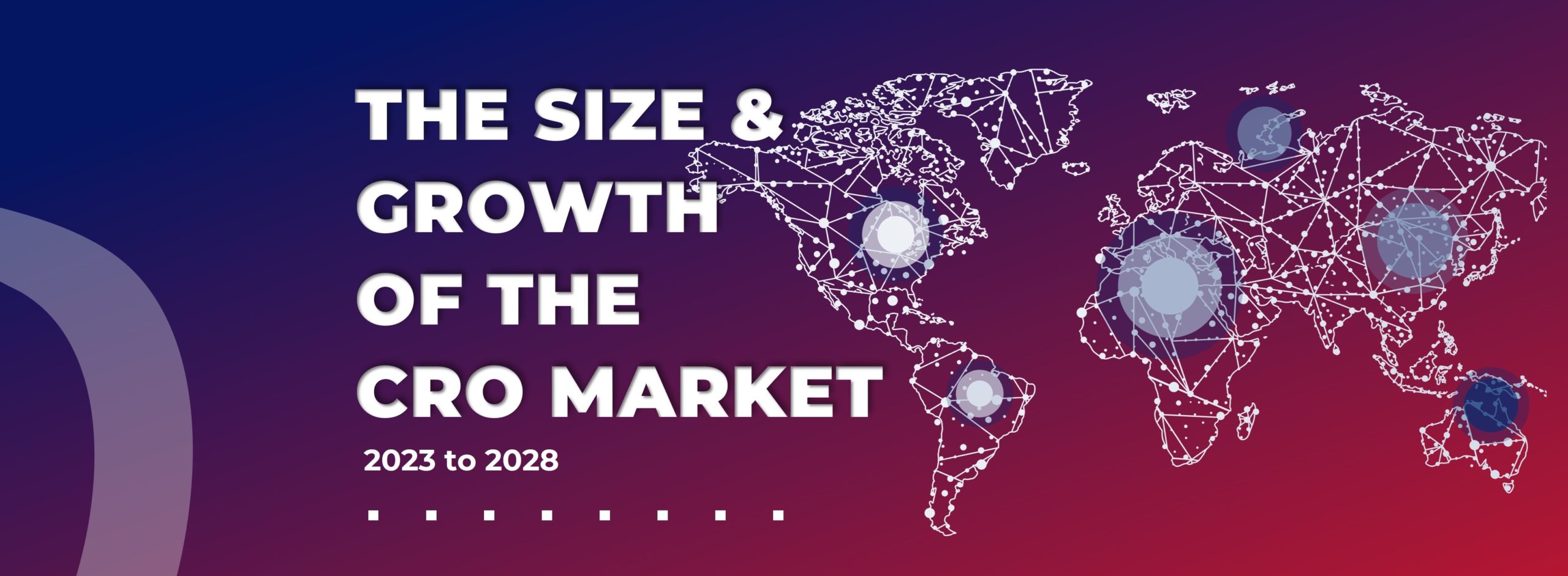
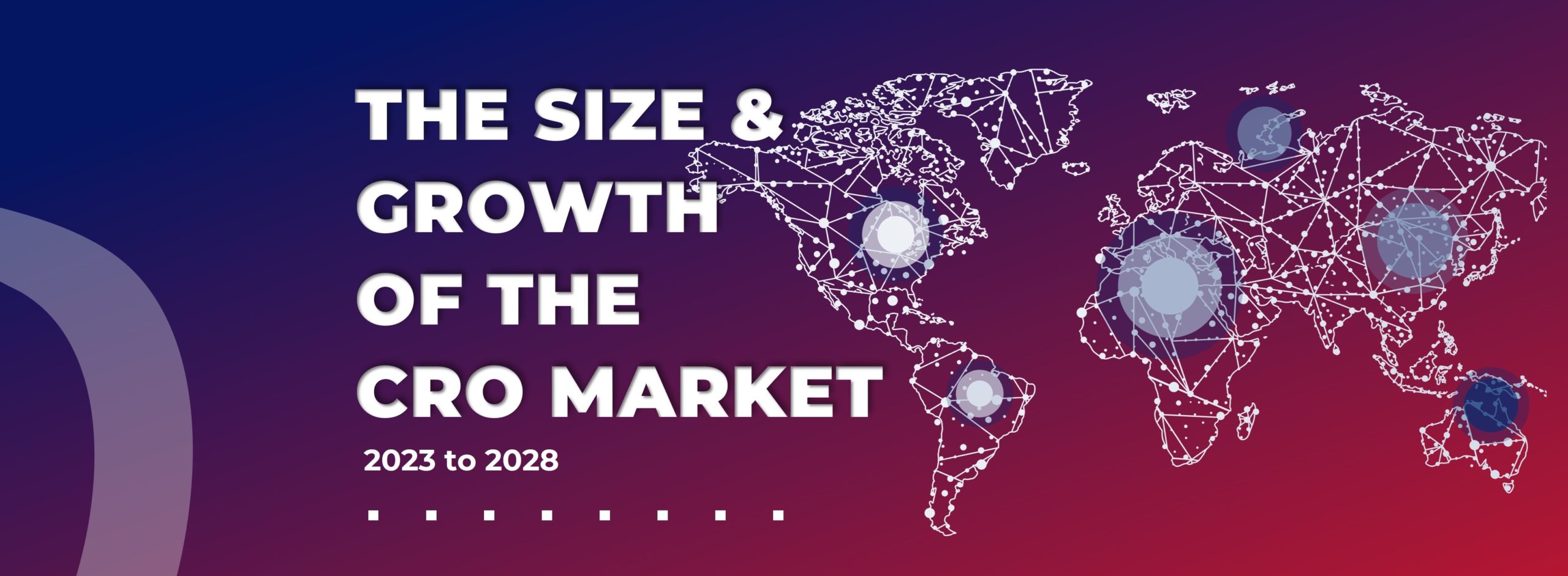
Clinical trial designs can be classified into several categories, each with its own unique characteristics and objectives. This guide provides a broad look at the many different types of clinical trial designs available, suggesting which designs are most suitable for specific research objectives and highlighting their key features, benefits, and considerations.
To digest the information more easily, we have divided this series on types of Clinical Trial Designs into 4 parts, each describing a set of clinical trial designs. This is Part 2 of 4. Please click here to read Part 1 of this series or fill out the form below to receive the full guide.
In case you wish to download this content, we made a PDF of this guide.
Download the PDF version of this guide now.
Stratified designs are similar to but distinct from block designs, though they are often confused with one another. Stratified designs are based on defining participants by some key characteristics (e.g., age or disease state) and controlling enrollment based on these factors. Frequently, the outcomes of each stratum are then compared. The goal of this design is to ensure that the sample for the study matches a pre-determined distribution of the stratification factors. Importantly, a stratification factor does not necessarily impact randomization or treatment assignment, though it may.
(The above refers to the strict definition of a stratified design, which is one that utilizes stratified sampling. Adding to the confusion between block designs and stratified designs, “stratified randomization” is a term that is largely analogous to block design.)
Example: A study stratified by region (U.S. or Europe) limits enrollment so that 50% of the sample are participants from the U.S. and 50% are participants from Europe; this stratification would not influence randomization to treatment at all unless the study was also a block design.
A factorial design study is a type of trial in which multiple interventions are tested simultaneously. Participants are randomized to different groups receiving various combinations of treatments. This design allows researchers to assess the impact of individual interventions as well as potential interactions between them. Factorial trials can efficiently evaluate multiple treatment strategies within a single study, saving time and resources. When planned and executed properly they can provide two or more studies’ worth of information in a single study but require care and reasonable confidence that treatments do not interact heavily. Frequently, “factorial design” typically refers to a full factorial design, where all possible combinations of interventions are assigned to participants. Partial or fractional factorial designs are ones in which not all possible combinations of interventions are assigned and must be very carefully planned to avoid imbalance or bias.
Example: A pain study that attempts to distinguish between Drug X and Drug Y for pain, as well as examining the effects when subjects are provided regular physiotherapy or not. Subjects can therefore be assigned to one of four conditions: Drug X + Physiotherapy; Drug Y + Physiotherapy; Drug X + No physiotherapy; Drug Y + No physiotherapy. This is a full factorial design.
Minimization randomization is a type of randomization technique that is somewhat similar to block designs. The goal of minimization randomization is to minimize the imbalance in each randomized arm based on pre-specified strata. When a new subject enters the study, the subject is hypothetically added to each arm, one at a time, and the imbalance score of that arm is calculated based on a pre-determined equation. The arms are then weighted so that the subject is more likely (or required) to be randomized to the arm(s) with the lowest imbalance score. This technique is particularly useful in small studies with several characteristics of interest where randomization alone may not be sufficient to achieve balance.
Example: A study is performing minimization randomization based on age (< 50, ≥ 50) and region (North America or South America). A new subject that is 60 years old and lives in South America is enrolled; the algorithm will temporarily assign the subject to each treatment arm, one at a time, and calculate an imbalance score. Generally (depending on the specific equation used), arms that have fewer subjects aged ≥ 50 and in South America will have lower imbalance scores, and it is more likely this subject will be randomized to those arms.
A crossover design study is a type of trial where the participants receive more than one intervention in different periods of time. Each participant receives each treatment in a predetermined order with a washout period between treatments. This design is particularly useful when studying chronic conditions or evaluating long-term interventions. Most frequently, this type of design is used to test a participant’s response on both an experimental intervention and a control; in this case, they are a type of Randomized Control Trial. Crossover trials can help minimize inter-patient variability and provide valuable insights into treatment effectiveness within individuals. The goal of this design is to determine the effects of the treatment over time and to control for individual differences.
A matched design study is another type of trial where the participants are matched based on their similarities in certain characteristics such as age or disease stage. Matched designs require there to be only two interventions; when one is a control, then they are a type of Randomized Control Trial. The matched participants are then randomly assigned such that the two pair members are on different interventions. These trials must be designed with care; how subjects are matched with one another can have a significant impact on the study results. Matching may be based on specific factors or on a more complex technique like propensity scores. This study design serves a similar purpose to a crossover design but can be used when a crossover design is not feasible. The goal of this design is to ensure that the groups are as similar as possible, reducing the potential for bias if the factors used for matching account for a good amount of inter-subject variability in treatment effect.
Matched design principles are also sometimes applied to retrospective or observational datasets, by creating the study sample out of pairs of closely matched subjects on different interventions in order to attempt to create “balanced” samples of each intervention from the data. How well this works to reduce bias is largely reliant on the matching method and how well the matching factors relate to the outcomes.
An enriched design study is a type of trial study enrollment is biased in favour of or restricted to individuals who are expected to benefit from the intervention being tested. This design is used to increase the efficiency of the study by focusing on the population most likely to benefit from the intervention. Frequently, enrichment is performed based on a biomarker (e.g. a cancer protein or genome known to be related to treatment effect) or on participant performance during a screening period (e.g. a study of pain medicine may not enroll subjects who respond too strongly to placebo during screening).
Example: A prior study shows that subjects who are age 60 or older appear to respond more strongly to Drug X. A new study uses an enriched design by enrolling mostly subjects who are age 60 or older.
A repeated measures design study is a type of trial where the same participants’ outcomes are measured multiple times over a period of time. This design is used to determine the effects of the intervention over time and to control for individual differences.
Repeated measures are useful by allowing the capture of more information from the same number of subjects; there are statistical models that can leverage the repeated measurements to provide more precise estimates of effects over time. Repeated measures are also important when studying time-to-event data in order to ensure that information about when events occur are sufficiently precise.
A crossover design is one example of a repeated measures design. Frequently, when “repeated measures” is brought up in common usage, it refers to designs where subjects’ measurements on one or more variables are captured on a regular and frequent basis (e.g. monthly). While, technically, a design where a variable is only measured twice (before and after treatment, or once on treatment A and once on treatment B) is a repeated measures design, the common use of the term implies more frequent assessment.
Example: Subjects on Drug X are being monitored for signs of cancer remission. Lesion size and RECIST evaluation grade are captured for each subject every two months until two years post-treatment or remission occurs.
In this part 2 of a 4 part series on the different types of clinical trial designs, we continue to explore how a variety of clinical trial designs can be used to generate reliable evidence about the safety and efficacy of new treatments.
The selection of a trial design should be based on the specific research objectives, the nature of the intervention being tested, the target population, available resources, and ethical considerations. Since each design has its own unique features, benefits, and considerations, study design decisions should be made collaboratively using input from trial stakeholders, subject matter experts, and statistical experts to not only understand the strengths and limitations of each, but to also ensure that the chosen design is the best fit for the goals of the study.
As medical knowledge advances, it is important for researchers to continue exploring innovative trial designs that adapt to emerging evidence, maximize efficiency and ultimately lead to improvements in overall patient care.
Providing 40 years of service, Alimentiv Statistics is a niche Contract Research Organization focused on Biostatistics, Statistical Analysis, Clinical Trial Design and Data Management. We invite you to see how we exceed our clients’ expectations.