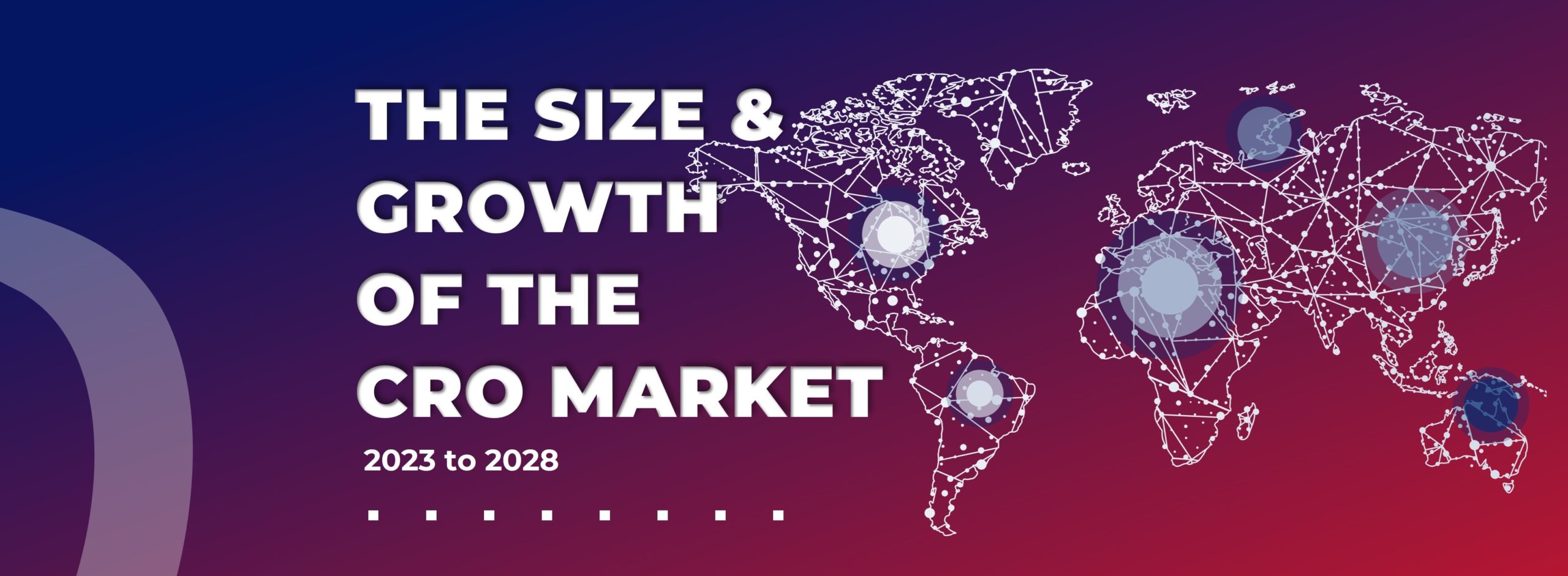
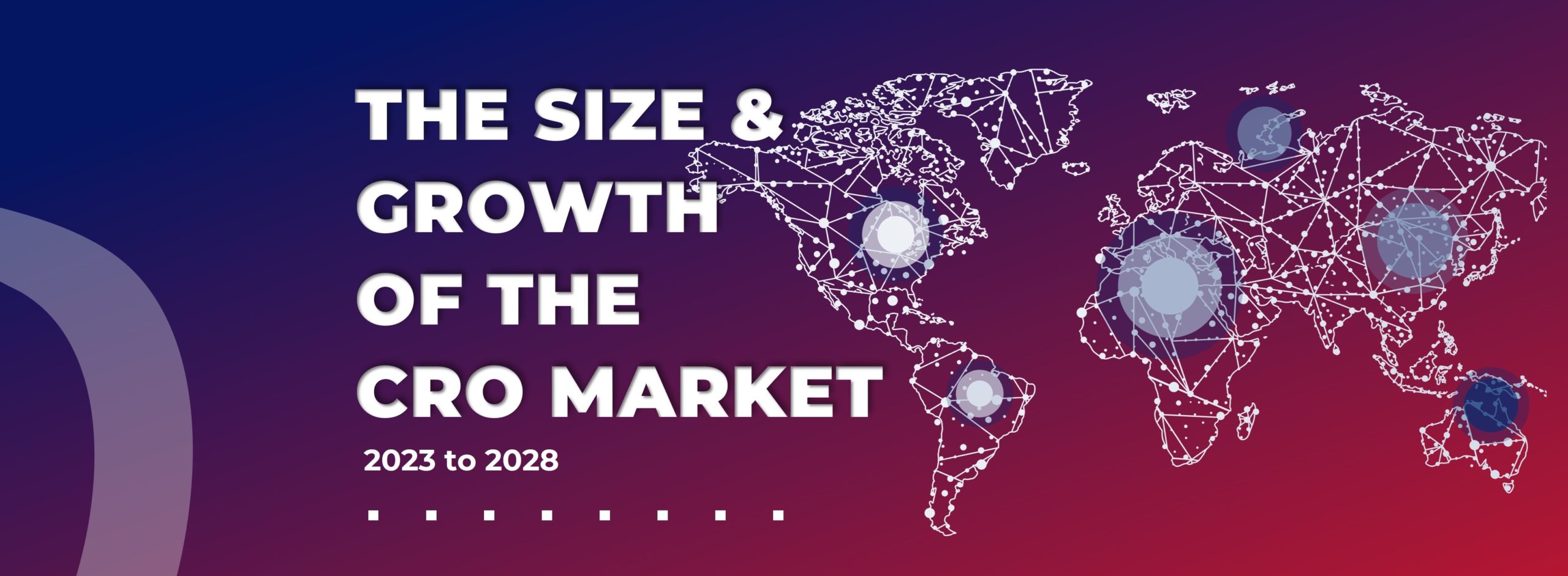
As we have seen in Parts 1, 2 and 3 of this series, clinical trial designs can be classified into several categories, each with their own characteristics and objectives. Part 4 of the guide continues to provide a broad look at the different types of clinical trial designs available, suggesting which designs are most suitable for specific research objectives and highlighting their key features, benefits, and considerations.
We have divided this series of types of Clinical Trial Designs into 4 parts, each describing a set of clinical trial designs. This is Part 4 of 4. Go here to view Part 1 and Part 2 here and Part 3 here.
In case you wish to download this content, we made a PDF of this guide.
Download the PDF version of this guide now.
An intent-to-treat population or a study design using the intent-to-treat principle is one in which all participants are analyzed according to the group to which they were originally assigned, regardless of whether they completed the study or remained on the assigned treatment or not. This design is used to control for dropouts, participants who do not adhere to treatment, sites that make treatment errors, and to ensure that the treatment groups are comparable.
Ideally, ITT consideration of subjects and data provides a less biased analysis of treatment effects, as it may reduce the possibility of bias introduced by treatment changes during the study. ITT helps keep the “fairness” of the original randomization by ignoring treatment crossover or dropout by subjects who are not responding to treatment.
True ITT analysis requires continuing to follow and measure subjects who withdraw from treatment or drop out of the study, which may pose difficulties. Ignoring issues with treatment adherence or protocol deviations may also obscure some effects; for instance, if a subject assigned to active treatment is accidentally given a placebo during the study, ITT analysis will underestimate the true effect of the treatment.
ITT analysis is often desired or required by regulatory authorities. Due to its drawbacks, however, it can also be important to define other study populations to allow for the examination of the data from alternate lenses. For example, safety analyses often consider subjects who have had any exposure to the active treatment under consideration as “active treatment” subjects to summarize adverse events.
Real-world data (RWD) is data collected outside of traditional clinical trial settings, such as electronic health records or patient-generated data. Real-world data are used in a study to generate real-world evidence on the safety and efficacy of treatments and interventions in a real-world setting.
RWD can be appealing since it may require fewer resources to collect than bespoke data from a study; however, many facets such as data collection and cleanliness need to be carefully considered to result in useful data.
Link to the FDA page on RWD/RWE:
https://www.fda.gov/science-research/science-and-research-special-topics/real-world-evidence
Real-world evidence (RWE) refers to evidence derived from real-world patient experiences, including their health outcomes, treatment patterns, and the impact of interventions. That is, RWE is the result of accumulating and analyzing RWD.
RWE is gaining prominence in the field of healthcare and research. It offers valuable insights into the effectiveness, safety, and value of medical interventions in real-world settings. Clinical trials, while essential, have limitations in terms of their controlled environments and selected patient populations. RWE provides a broader understanding of how treatments and interventions perform in diverse patient populations, beyond the controlled settings of clinical trials. RWE helps identify gaps in knowledge, inform treatment guidelines, and support healthcare policies that optimize patient outcomes.
RWE can be obtained by analyzing real-world data from a wide range of sources. Electronic health records (EHRs) capture patient data during routine clinical encounters, offering valuable insights into treatment patterns and outcomes. Claims databases contain information on diagnoses, procedures and prescriptions, providing a comprehensive overview of patient care. Patient registries focus on specific diseases or conditions, tracking long-term outcomes and treatment effectiveness. Additionally, wearable devices and mobile health apps generate real-time data on patient behaviours and health indicators. All of these sources provide RWD that can be organized, cleaned, and analyzed to create RWE.
Link to the FDA page on RWD/RWE:
https://www.fda.gov/science-research/science-and-research-special-topics/real-world-evidence
A parallel-group (or parallel-arm) trial is a common research design used in clinical trials. It involves the random assignment of participants into two or more groups, each receiving a different intervention or treatment. Often there will be at least one experimental group that receives the treatment being tested, and at least one control group that receives a placebo or standard of care treatment. The outcomes of the randomly assigned treatments are then analyzed, usually by comparing results between arms.
Randomization is a crucial aspect of parallel-group trials. It ensures that participants are assigned to intervention groups in a random and unbiased manner, reducing the potential for confounding variables. Randomization generally helps achieve a balanced distribution of participant characteristics across groups, increasing the reliability of the study’s findings.
The purpose of parallel-group trials is to assess the efficacy, safety, or other relevant outcomes of each intervention in a controlled and scientifically rigorous manner.
Parallel-group trials offer several advantages in clinical research. First, they provide a robust design for comparing the effectiveness of different interventions. Second, randomization helps minimize bias and confounding variables, enhancing the internal validity of the study. Third, parallel-group trials can be conducted in various settings, making them applicable to different research scenarios.
However, a limitation of this type of trial is the potential for dropout or non-compliance among participants, which can affect the validity of the study results. Additionally, parallel-group trials may not be suitable for evaluating certain interventions, such as those with long-term or cumulative effects. Finally, parallel-group trials may not be the most efficient or powerful design for many settings and may require larger sample sizes.
Cluster-randomized trials have emerged as a robust methodology to ensure research validity in scenarios where individual randomization is either impractical or ethically unviable. In a cluster-randomized trial, participants are grouped into clusters—such as geographical regions, healthcare facilities, or educational institutions—and then each cluster is randomized (e.g. an entire geographical region would be randomized to a single treatment for all participants in that region). By grouping and randomizing participants in this manner, researchers can attain a more holistic perspective on the effects of interventions and can overcome obstacles that impede the traditional approach. This powerful design not only ensures methodological rigour but also opens up new avenues for conducting impactful studies.
One primary advantage of cluster-randomized trials is their feasibility when dealing with real-world settings. In certain scenarios, it may be logistically challenging or ethically inappropriate to randomize individuals. Other advantages such as reduced contamination and optimized statistical power also make cluster-randomized trials a valuable approach.
An N-of-1 trial is a type of trial in which a single patient receives a series of interventions in a randomized, crossover design. This design is used to determine the best treatment for an individual patient. Unlike traditional clinical trials that involve a group of participants, N-of-1 trials focus on a single patient. An N-of-1 trial is a refocusing from a treatment’s effect on a general or “average” population and instead a narrowing of focus to treat an individual patient’s well-being and health as the primary concern of the trial.
In an N-of-1 trial, the patient serves as their own control, meaning they undergo a series of treatment periods where they receive both the experimental treatment and a placebo or alternative treatment in a randomized and blinded manner. The treatment periods are typically repeated multiple times to gather sufficient data for analysis.
These trials aim to provide personalized evidence regarding the effectiveness of a treatment for a particular patient. They are particularly useful in cases where individual variation in treatment response or rare conditions make it challenging to apply generalized research findings to an individual’s specific situation. The data collected from N-of-1 trials can help guide treatment decisions by providing detailed information about how an individual responds to a specific intervention.
Systematic reviews and meta-analyses are research methods used to synthesize and analyze existing scientific literature on a particular topic, providing a comprehensive and robust summary of the available evidence.
A systematic review involves a rigorous and structured approach to identify, select, and critically appraise relevant studies that address a specific research question. Researchers systematically search multiple databases, screen and assess the quality of studies, extract data and analyze the findings. By using predefined criteria and transparent methodology, systematic reviews aim to minimize bias and provide a useful overview of the available evidence.
Meta-analysis is a statistical technique used to combine the results from multiple studies included in a systematic review. It involves pooling the data from individual studies to calculate summary effect sizes and estimating the overall treatment effect or association between variables. Meta-analysis can increase statistical power and precision by analyzing a larger sample size than any single study, which can lead to more reliable and generalizable conclusions.
By synthesizing the findings of multiple studies, these methods may enable researchers and practitioners to draw more robust conclusions about the effectiveness of interventions, the prevalence of conditions, or the associations between variables.
It’s important to note that the quality of a systematic review and meta-analysis heavily depends on the quality of the included studies, the rigour of the methodology and the transparency of reporting. Well-conducted systematic reviews and meta-analyses follow established guidelines and adhere to strict methodological standards to ensure the reliability and validity of findings.
Non-inferiority trials are a type of clinical research study designed that tries to determine whether a new treatment is not significantly worse than an established or standard treatment by a predefined margin. This is in contrast to traditional superiority trials, which aim to demonstrate that a new treatment is superior to an existing one. A non-inferiority approach is often used in situations where demonstrating superiority over an established treatment may be challenging due to ethical concerns, high cost, or practical limitations.
Researchers conduct non-inferiority trials by first defining a non-inferiority margin or the maximum difference they consider clinically acceptable. After the trial is complete, statistical analyses are then performed to determine whether the new treatment falls within this margin compared to the standard treatment. If the new treatment is shown within the bounds of statistical significance to have a treatment effect that is not less effective than the standard treatment by more than the non-inferiority margin, then it is considered non-inferior.
Non-inferiority trials are relevant when evaluating new interventions that may offer additional benefits such as improved safety profiles, ease of administration or cost-effectiveness, helping to expand treatment options, and guiding clinical decision-making, but may not be superior to the standard treatment or would require a prohibitively large study to show superiority. They are also useful in situations where it may not be ethical to conduct traditional superiority trials.
In part 4 of a 4-part series on the different types of clinical trial designs, we continue to explore how a variety of clinical trial designs can be used to generate reliable evidence about the safety and efficacy of new treatments.
As previously stated in parts 1,2 and 3, the selection of a trial design should be based on the specific research objectives, the nature of the intervention being tested, the target population, available resources, and ethical considerations. Since each design has its own unique features and considerations, study design decisions should be made collaboratively using input from trial stakeholders, subject matter experts, and statistical experts to not only understand the strengths and limitations of each but to also ensure that the chosen design is the best fit for the goals of the study.
As medical knowledge advances, it is important for researchers to continue exploring innovative trial designs that adapt to emerging evidence, maximize efficiency and ultimately lead to improvements in overall patient care.
Providing 40 years of service, Alimentiv Statistics is a niche Contract Research Organization focused on Biostatistics, Statistical Analysis, Clinical Trial Design and Data Management. We invite you to see how we exceed our clients’ expectations.